Federated AI Ecosystem
Collaborative Learning and Knowledge Transfer with Data Protection
Why FedAI?
FedAI is a community that helps businesses and organizations build AI models effectively and collaboratively, by using data in accordance with user privacy protection, data security, data confidentiality and government regulations.
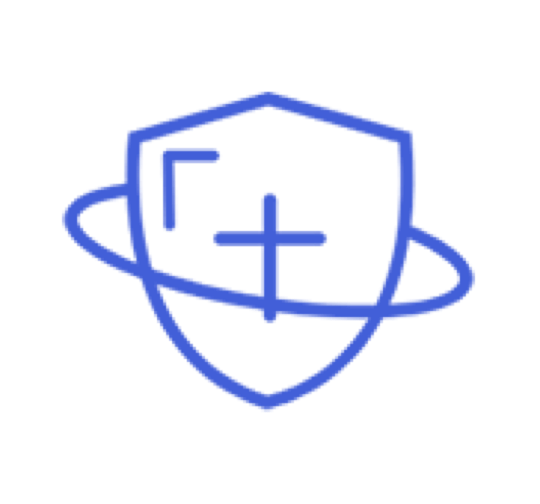
Security & Compliance
Meet security and
compliance requirements;
Ensure data privacy and
model security

Joint & Expansion
of various industries;
Exploit and extend
the value of data

Promotion & Empowerment
Sustainable and intelligent
incentive mechanisms;
Stable and win-win
business ecosystem
Our Research
Hankz Hankui Zhuo, Wenfeng Feng, Qian Xu, Qiang Yang, Yufeng Lin
SecureBoost: A Lossless Federated Learning Framework
Kewei Cheng, Tao Fan, Yilun Jin, Yang Liu, Tianjian Chen, Qiang Yang
Federated Machine Learning: Concept and Applications
Qiang Yang, Yang Liu, Tianjian Chen, Yongxin Tong
The 1st International Workshop on Federated Machine Learning for User Privacy and Data Confidentiality (FML 2019)
Salon: Webank & DataFun (Chinese Version Only)
打破数据孤岛:联邦学习技术实践探索
CCF-TF Talk No.14 (Chinese Version Only)
联邦学习(Federated Machine Learning)技术及数据隐私保护
Open Source Projects
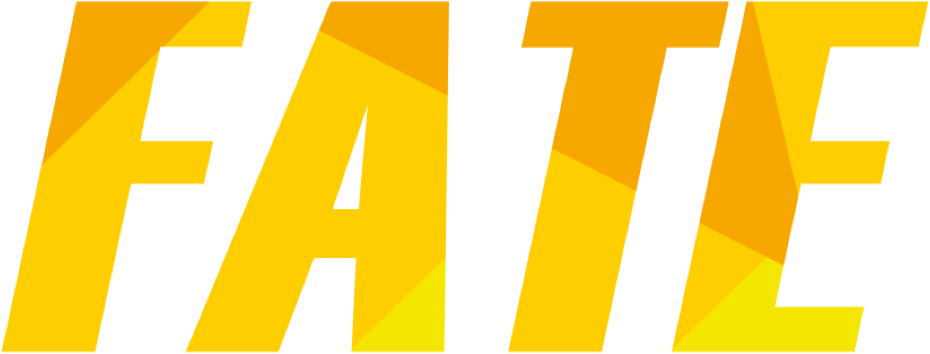
FATE
FATE (Federated AI Technology Enabler) is an industrial grade framework designed to support Federated Learning architectures and secure computation of ANY machine learning algorithms.
EggRoll
Eggroll is a distributed infrastructure with a unity of computing, storage and communication targeted at large-scale machine learning and deep learning applications.
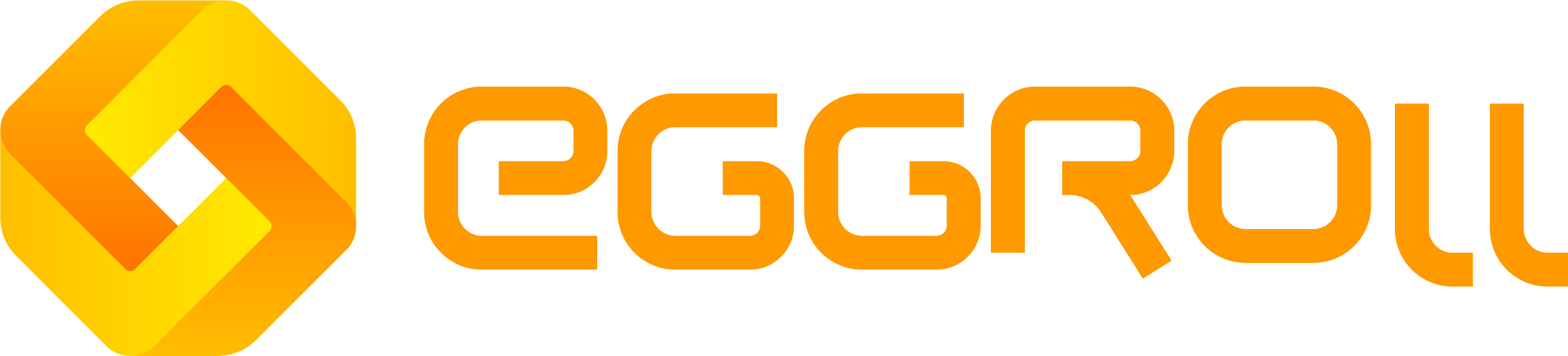